While the other rat will keep moving and starve.
Ex. 1: A married couple decide to have children till they have a boy and then to
Stop. (i) Is the process regulatory ? (ii)What is the matrix of transition prob-
Abilities ?
Ex. 2: Is the game “Heads, I win; Tails, we toss again” regulatory?
So far we have considered only the way in which a Marko-
Vian machine moves to its goal. In principle, its sole difference from
A determinate machine is that its trajectory is not unique. Provided
We bear this difference in mind, regulation by the Markovian
Machine can have applied to it all the concepts we have developed
In the earlier chapters of this Part.
The warning given in S.11/11 (pare. 5) must be borne in mind.
The steps that take a Markovian machine along its trajectory are
Of a smaller order of magnitude than the steps that separate one act
Of regulation (one “move” in the sense of S.11/3) from another.
The latter steps correspond to change from one trajectory to
Another — quite different to the change from one point to the next
Along one trajectory.)
Thus the basic formulation of S.11/4 is compatible with either
Determinate or Markovian machines in T and R to provide the
Actual outcome. No difference in principle exists, though if we
Describe their behaviour in psychological or anthropomorphic
Terms the descriptions may seem very different. Thus if R is
Required (for given disturbance) to show its regulatory power by
Going to some state, then a determinate R will go to it directly, as
If it knows what it wants, while a Markovian R will appear to
Search for it.
The Markovian machine can be used, like the determinate, as a
Means to control; for the arguments of S.11/14 apply to both (they
Were concerned only with which outcomes were obtained, not
With how they were obtained.) So used, it has the disadvantage of
Being uncertain in its trajectory, but it has the advantage of being
Easily designed.
232
Regulation by error. The basic formulation of S 11/4 is of
Extremely wide applicability. Perhaps its most important particular
Case occurs when both T and R are machines (determinate or Mark-
|
|
Ovian) and when the values of E depend on the various states of
equilibrium that T may come to, with , η as some state (or states)
That have some appropriate or desired property. Most physical reg-
Ulators are of this type. If R and T are Markovian machines the
bringing of T to a desired state of equilibrium η by the action of R
Can readily be achieved if advantage is taken of the fundamental
Fact that if two machines (such as T and R are now assumed to be)
Are coupled, the whole can be at a state of equilibrium only when
Each part is itself at a state of equilibrium, in the conditions pro-
Vided by the other. The thesis was stated in S.5/13 for the determi-
Nate machine, but it is just as true for the Markovian.
Let the regulator R be built as follows. Let it have an input that
can take two values, β and γ. When its input is β (for “bad”) let no
state be one of equilibrium, and when its input is γ (for “good”) let
Them all be equilibrial. Now couple it to T so that all the states in
η are transformed, at R’s input, to the value γ, and all others to the
value β. Let the whole follow some trajectory. The only states of
Equilibrium the whole can go to are those that have R at a state of
Equilibrium (by S.5/13); but this implies that R’s input must be at
γ, and this implies that T’s state must be at one of η. Thus the con-
Struction of R makes it a vetoer of all states of equilibrium in T
save those in η. The whole is thus regulatory; and as T and R are
Here Markovian, the whole will seem to be hunting for a “desira-
Ble” state, and will stick to it when found. R might be regarded as
“directing” T’s hunting.
The possibility that T and R may become trapped in a stable
region that contains states not in η can be made as small as we
Please by making R large, i.e. by giving it plenty of states, and by
|
|
seeing that its β − matrix is richly connected, so that from any state
It has some non- zero probability of moving to any other state.)
Ex. 1: What, briefly, must characterise the matrix γ, and what β?
*Ex. 2: Show that the thesis of S.5/13 is equally true for the Markovian machine.
The homeostat. In this form we can get another point of view
Дата добавления: 2019-11-16; просмотров: 216; Мы поможем в написании вашей работы! |
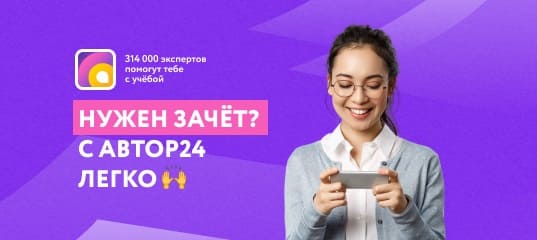
Мы поможем в написании ваших работ!