Achieving energy-efficient computation in a post Moore’s law world
Another multiple order of magnitude discrepancy between biological and artificial systems lies in their energy expenditure. The human brain spends only 20 watts of power, while supercomputers operate in the megawatt range. In this sense, we are all literally dimmer than light bulbs! A key reason for this discrepancy likely lies in an over-reliance on digital computing itself. While the digital revolution has powered the rise of modern information technology, it may now be thought of as a suboptimal legacy technology in our forward-looking quest for achieving artificial intelligence. The reason is that digital computation requires flipping every bit at intermediate stages of a computation with extremely high reliability. However the laws of thermodynamics then exact a considerable energetic cost for every fast and reliable bit flip [17], thereby precluding high energy efficiency.
In contrast, biological computation using molecules within cells, as well as neurons within brains, looks astoundingly noisy and imprecise. However, every intermediate step of a biological computation is just reliable enough for the final answer to be just good enough. Moreover, the brain intelligently up or down regulates energy costs according to the desired speed of communication (something our mobile phone processors are just starting to do). For example, consider the cost of a single bit in the brain as it travels through a target neuron [18]. It starts off as the stochastic release of a vesicle, whose contents diffuse across the space between the source neuron and target neuron at a speed of 1 millimeter per second, burning only 2.3 femto-Joules (fJ). This slow speed is fine because the space between neuronal connections is only 20 nanometers. This chemical signal gets converted to a passive electrical signal that flows through the neuron cell body at a speed of 1 meter per second, burning 23 fJ to traverse about 10 micrometers. Finally, it reaches the axon terminal and gets converted to a spike, which travels 100 meters per second along the axon, burning 6000 fJ to travel 1 cm. Thus in going from chemical to passive electrical signalling, the brain dynamically upregulates communication speed by a factor of 1000 to traverse distances that increase by a factor of 1000, incurring a 10-fold increase in energy expenditure. Similarly, in going from passive to active electrical signalling, the brain increases communication speed by a factor of 100 to traverse distances that increase by a factor of 1000, incurring about a 200-fold increase in energy expenditure.
|
|
Thus the brain spends more energy only when more speed is actually needed and only when more reliability is required. In contrast, digital computers operate on a rigid synchronous clock, and at each clock tick many transistors must reliably flip state. In summary, the apparent chaos of biological computation need not be unavoidable messiness, but rather may reflect desirable principles of highly energy efficient design. To achieve such efficiency in our AI hardware, it may be essential to follow such principles of biological computation.
AI for neuroscience and neuroscience for AI: a virtuous scientific spiral
Recent exciting developments in the interaction between neuroscience and AI involve the development of deep and recurrent neural network models as models for different brain regions of animals performing tasks. This approach has achieved success for example in the ventral visual stream [19], auditory cortex [20], prefrontal cortex [21], motor cortex [22], and retina [23,24]. In many of these cases, when a deep or recurrent network is trained to solve a task, its internal representations look strikingly similar to the internal neural activity patterns measured in an animal trained to solve the same task. Thus we obtain often highly complex yet surprisingly veridical models of the operation of different brain regions during different tasks, raising a fundamental question: how do we understand what these models are doing and how they work? More precisely, how does the learned network connectivity and neural dynamics generate task performance? AI currently faces the same problem in understanding what it’s neural models are actually doing. While some engineers argue that it is not necessary to understand how neural networks work - it only matters that they do work well - it is nevertheless likely that a deeper scientific understanding of how the successes and failures of current networks arise out of their connectivity and dynamics will subsequently lead to improved networks. Indeed, hardly ever in the history of the interaction between science and technology has a deeper scientific understanding not lead to better technology. Moreover, in certain applications of AI, especially in medical diagnosis or law, explainable or interpretable AI is essential to widespread adoption. For example, doctors and judges would be loathe to use the recommendations of AI systems on their cases if they could not understand why these systems made the decisions they did.
|
|
Thus both neuroscience and AI have deeply shared scientific goals of understanding how network performance and decision making arises as an emergent property of network connectivity and dynamics. Therefore the development of ideas and theories from theoretical neuroscience, and applied physics and mathematics could help in analyzing AI systems. Moreover, the behavior of AI systems could change the nature of experimental design in neuroscience, focusing the experimental effort on those aspects of network function that are poorly understood in AI. Overall, there is much to be gained from tighter connections between neuroscience, AI, and many other theoretical disciplines, which could bring about unified laws for the emergence of intelligence in biological and artificial systems alike, as we suggest next.
Дата добавления: 2019-02-26; просмотров: 173; Мы поможем в написании вашей работы! |
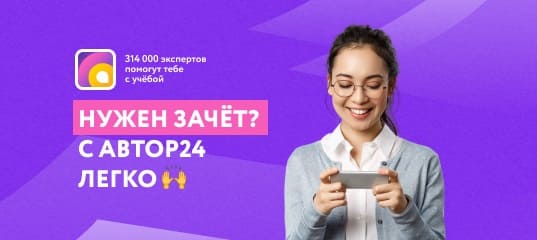
Мы поможем в написании ваших работ!